key words:
GNN, Design layouts, Human-machine collaboration
Year:
2022
Abstract: From sketch concepts to concrete design layouts, the deep learning network learns 3000 examples of architectural layouts. The architect only needs to input the sketch design intent and conditional constraints. The deep learning network then lays out a layout that meets the architect’s preferences and constraints based on the database. The data shows that we are able to predict building layouts with 96% accuracy (40% masking rate) after only 16 rounds of training.
Our findings demonstrate the efficacy of our co-design framework in accurately reasoning about 3D voxel models while providing architects with control over the design process. This research makes a new contribution to the human-computer framework in 3D building design by enabling architects to input local constraints and explore entire 3D building shapes and internal spatial partitions directly in 3D space. It highlights the potential of GNN to improve the accuracy and architectural control of 3D generated models. Our work reflects a promising direction for solving the challenges of 3D building shape exploration and spatial partitioning by allowing architects and AI to collaborate to flexibly create 3D models that meet complex site constraints and architect preferences in a 3D spatial network. Building-GNN acts as a partner to rapidly complete detailed reasoning and provide concrete prototypes to accommodate architects’ feedback. It utilises AI to accelerate in the design process not only the architect’s perception and understanding of the site, which is difficult for machines to surpass, to assist the AI in interactively exploring the 3D building layout results. The feedback loop process between the architect and the AI has the potential to facilitate a tighter, more creative and flexible design process.
| CONCEPT |
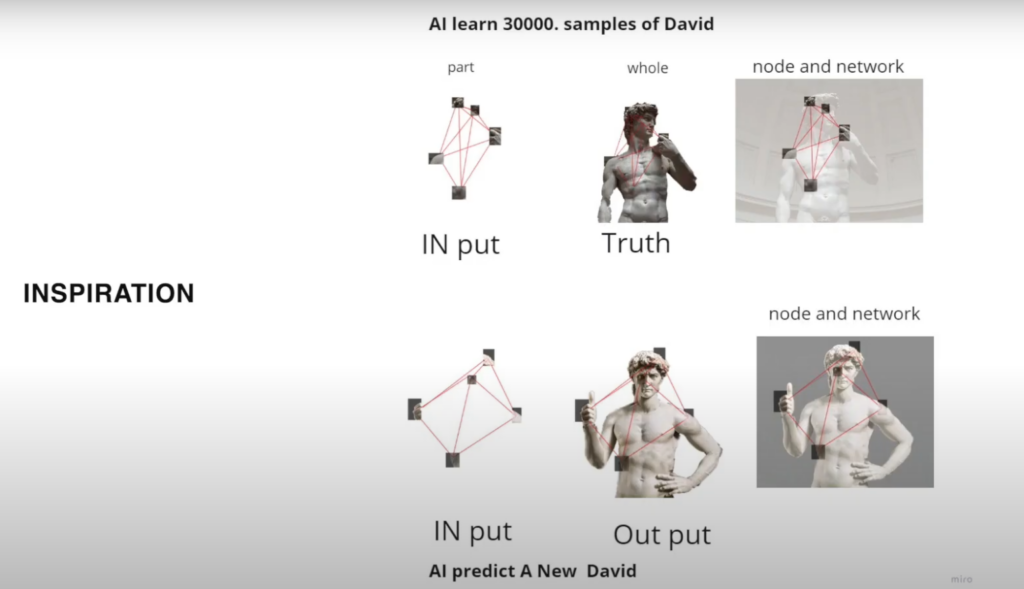
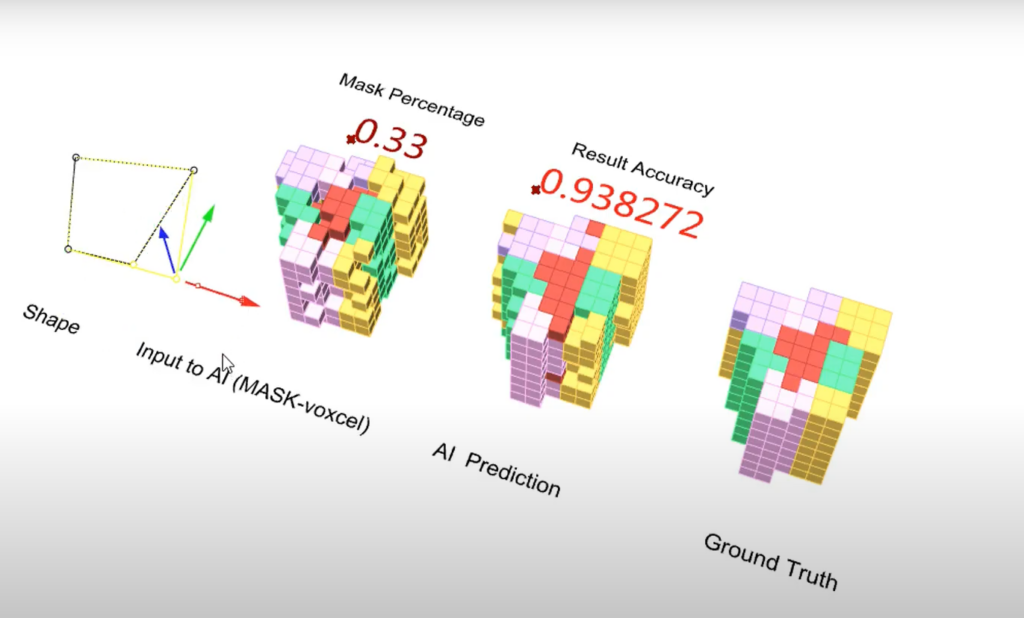
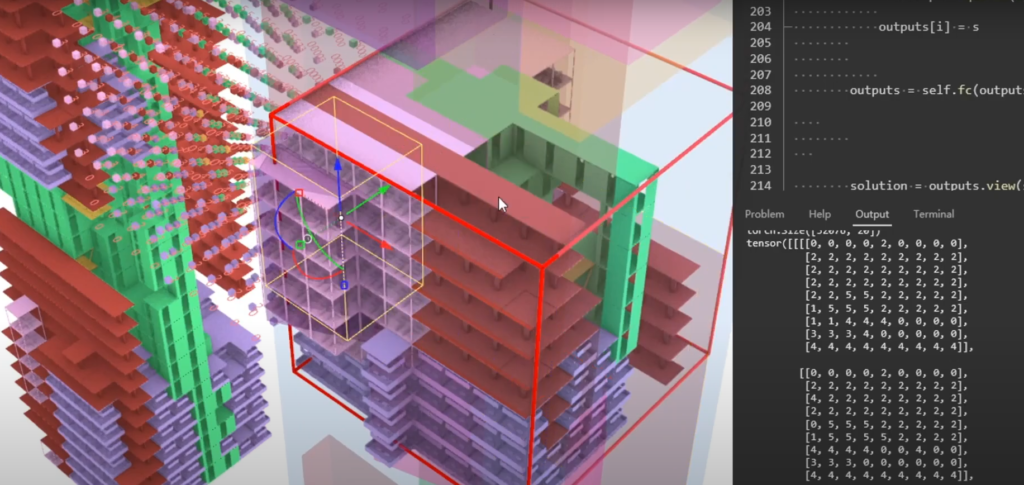
| APPLY SCENARIOS |

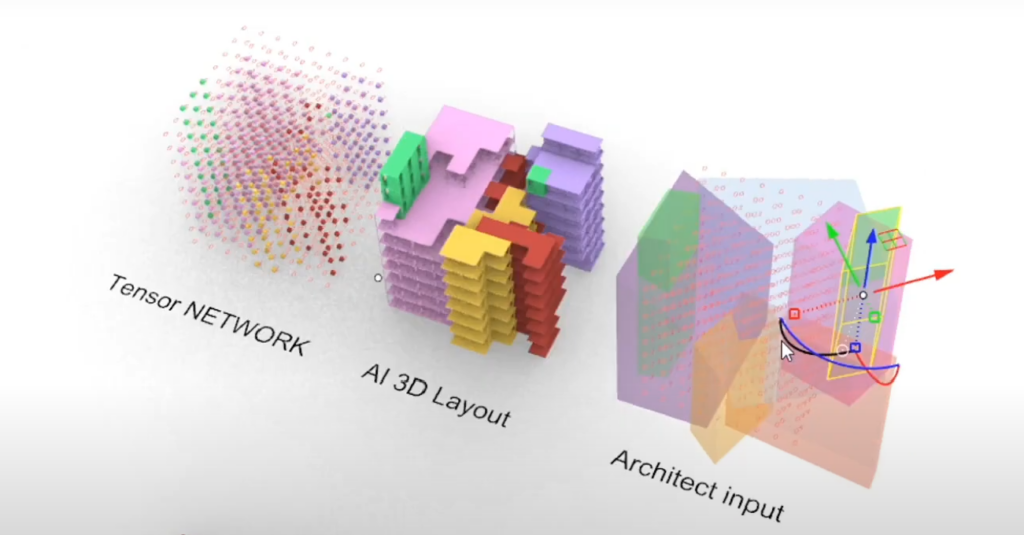

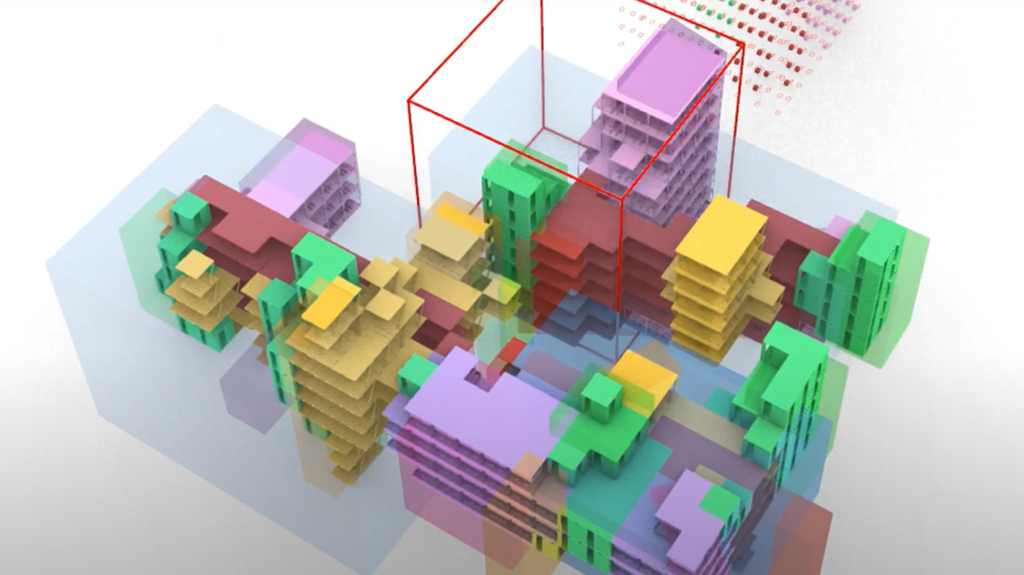

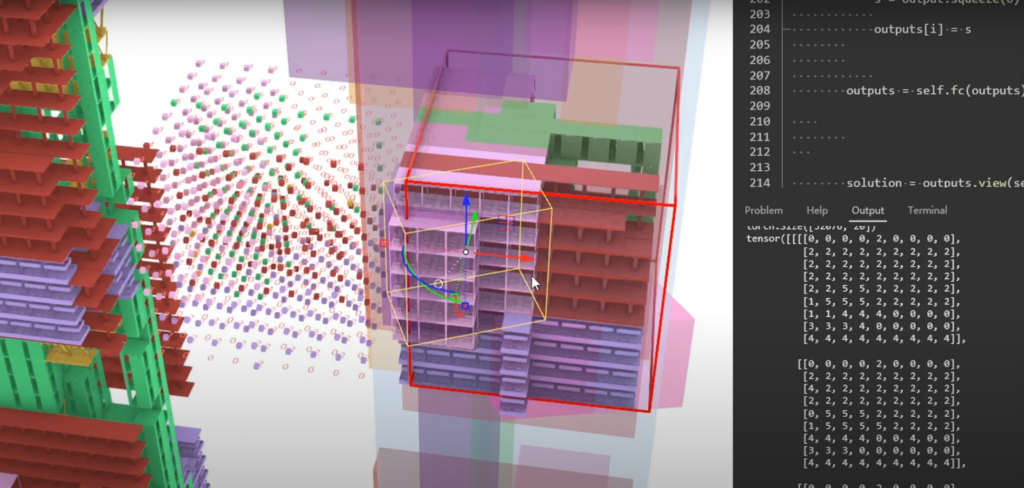